
异常检测算( OneClassSVM、EllipticEnvelope、IsolationForest、LocalOutlierFactor)法比较,基于四个仿真数据集
发布日期:2021-06-29 05:44:50
浏览次数:2
分类:技术文章
本文共 640 字,大约阅读时间需要 2 分钟。
异常检测算( OneClassSVM、EllipticEnvelope、IsolationForest、LocalOutlierFactor)法比较,基于四个仿真数据集
This example shows characteristics of different anomaly detection algorithms on 2D datasets. Datasets contain one or two modes (regions of high density) to illustrate the ability of algorithms to cope with multimodal data.
# 使用数据生成模块生成四个仿真数据集(两维数据集)。数据集包含一个或者两个团(密度富集区)来考察模型应对多模态(多峰)数据的能力;
For each dataset, 15% of samples are generated as random uniform noise. This proportion is the value given to the nu parameter of the OneClassSVM and the contamination parameter of the other outlier detection algorithms. Decision boundaries between inliers and outl
转载地址:https://blog.csdn.net/zhongkeyuanchongqing/article/details/117522900 如侵犯您的版权,请留言回复原文章的地址,我们会给您删除此文章,给您带来不便请您谅解!
发表评论
最新留言
能坚持,总会有不一样的收获!
[***.219.124.196]2024年04月10日 17时40分51秒
关于作者
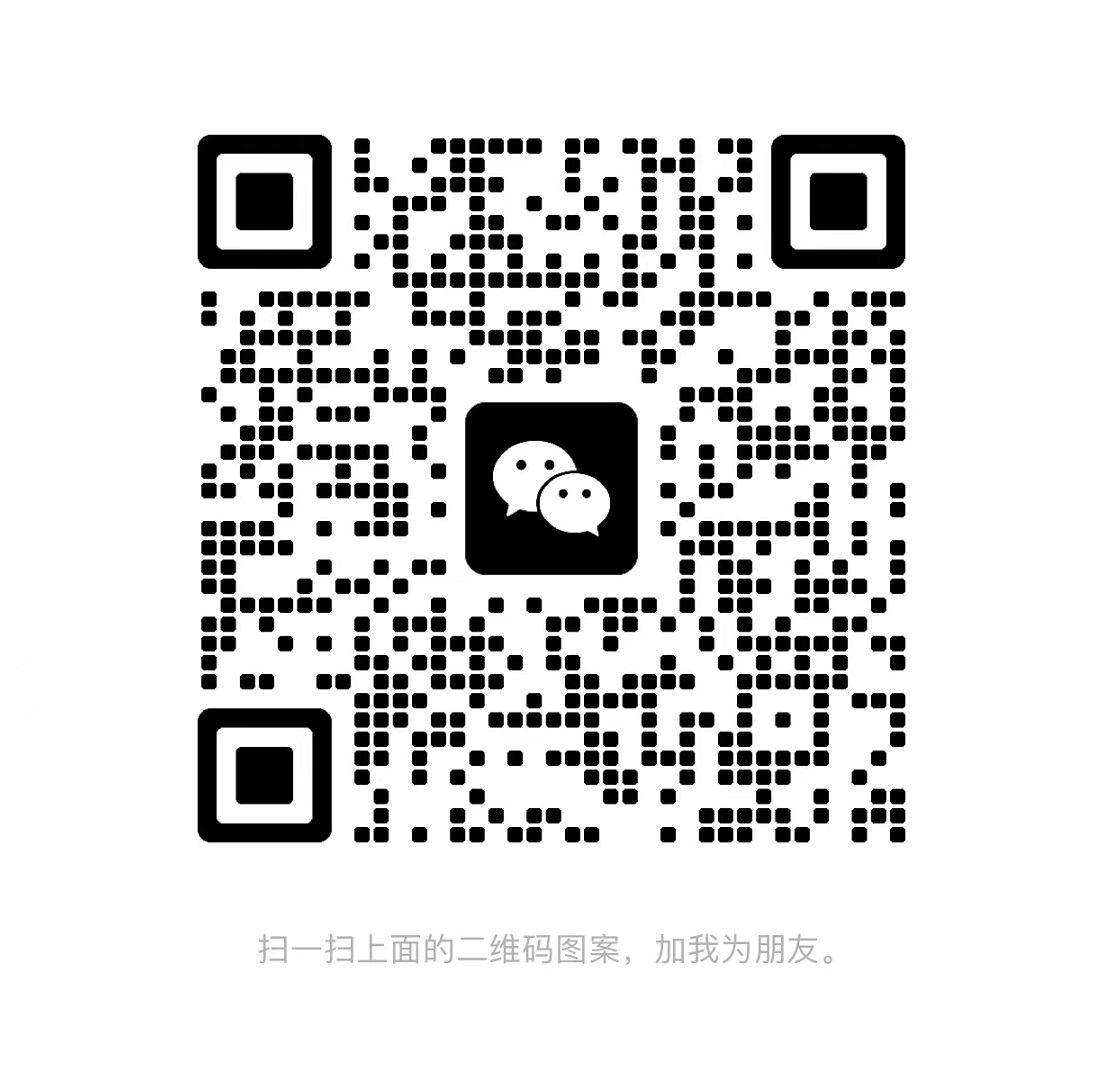
喝酒易醉,品茶养心,人生如梦,品茶悟道,何以解忧?唯有杜康!
-- 愿君每日到此一游!
推荐文章
kangle https设置
2019-04-29
Linux下EasyPanel版本安装及升级
2019-04-29
raspberry pi(树莓派) + easycap d60 视频采集
2019-04-29
WebRTC
2019-04-29
rfc5766-turn-server NAT
2019-04-29
webrtc详细教程
2019-04-29
Android IOS WebRTC 音视频开发总结
2019-04-29
报表图表样式
2019-04-29
支付宝
2019-04-29
Android开发资源收集
2019-04-29
android模板图例
2019-04-29
树莓派网线直连
2019-04-29
复合材料培训(I第七期)
2019-04-29
复合材料生活中的应用
2019-04-29
ABAQUS复合材料(适合小白)
2019-04-29
ABAQUS高级案例解析
2019-04-29
人工智能药物研发
2019-04-29
【超级干货+福利】AIDD最全面的学习教程
2019-04-29
最新通知:AIDD与网络药理学资料大全
2019-04-29