
本文共 1070 字,大约阅读时间需要 3 分钟。
目标检测中bbox回归中class-agnostic和class-specific的区别在哪?
-
class-specific 方式,很多地方也称作class-aware的检测,是早期Faster RCNN等众多算法采用的方式。它利用每一个RoI特征回归出所有类别的bbox坐标,最后根据classification 结果索引到对应类别的box输出。这种方式对于ms coco有80类前景的数据集来说,并不算效率高的做法。
-
class-agnostic 方式只回归2类bounding box,即前景和背景,结合每个box在classification 网络中对应着所有类别的得分,以及检测阈值条件,就可以得到图片中所有类别的检测结果。当然,这种方式最终不同类别的检测结果,可能包含同一个前景框,但实际对精度的影响不算很大,最重要的是大幅减少了bbox回归参数量。
-
For a class-aware detector, if you feed it an image, it will return a set of bounding boxes, each box associated with the class of the object inside (i.e. dog, cat, car). It means that by the time the detector finished detecting, it knows what type of object was detected.
-
For class-agnostic detector, it detects a bunch of objects without knowing what class they belong to. To put it simply, they only detect “foreground” objects. Foreground is a broad term, but usually it is a set that contains all specific classes we want to find in an image, i.e. foreground = {cat, dog, car, airplane, …}. Since it doesn’t know the class of the object it detected, we call it class-agnostic.
本文转载自:
转载地址:https://liumin.blog.csdn.net/article/details/117409117 如侵犯您的版权,请留言回复原文章的地址,我们会给您删除此文章,给您带来不便请您谅解!
发表评论
最新留言
关于作者
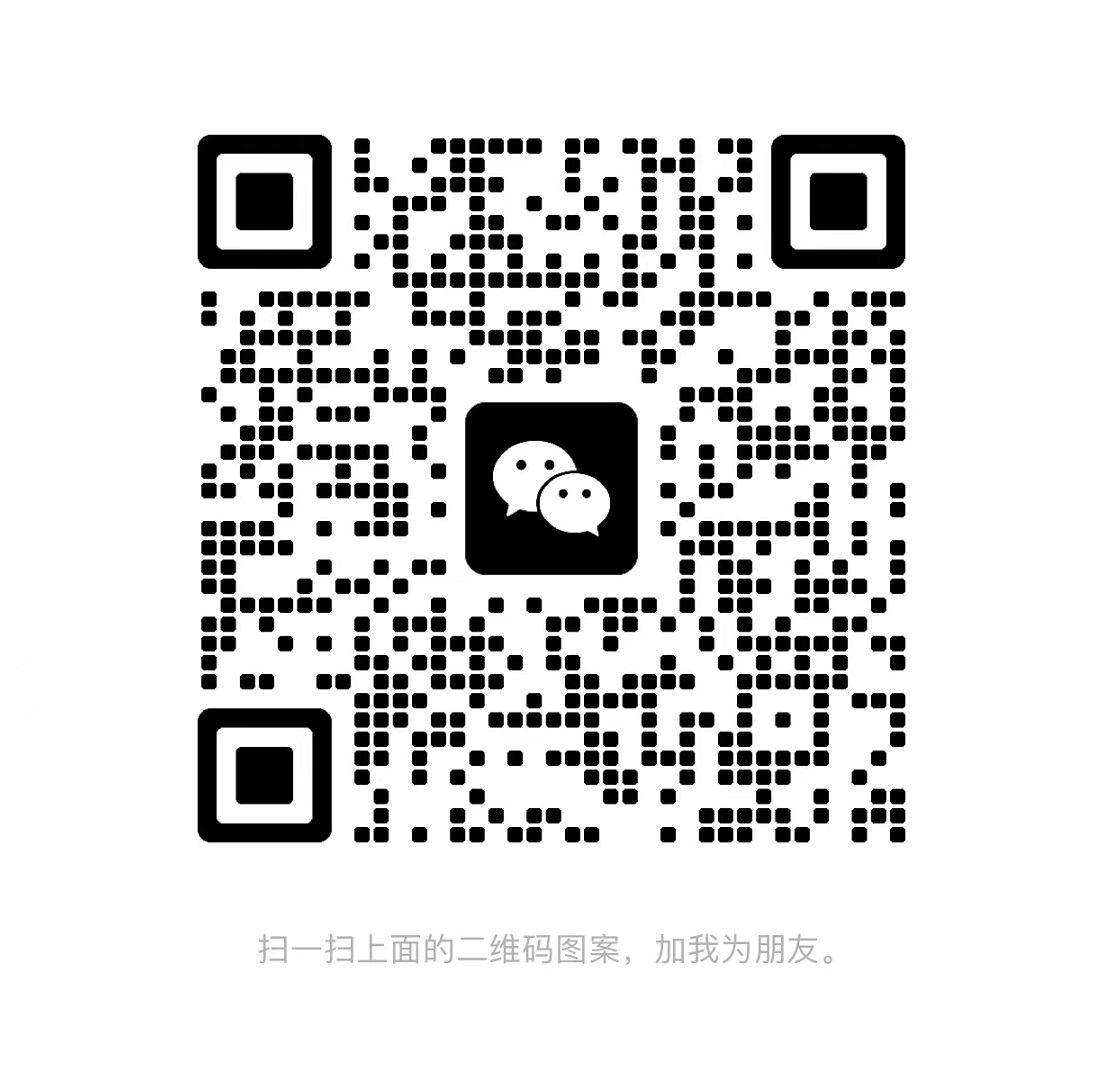