
LeetCode实战:合并两个有序链表
发布日期:2021-06-30 22:56:53
浏览次数:2
分类:技术文章
本文共 1112 字,大约阅读时间需要 3 分钟。
题目英文:
Merge two sorted linked lists and return it as a new list. The new list should be made by splicing together the nodes of the first two lists.
Example:
Input: 1->2->4, 1->3->4
Output: 1->1->2->3->4->4
题目中文:
将两个有序链表合并为一个新的有序链表并返回。新链表是通过拼接给定的两个链表的所有节点组成的。
示例:
输入:1->2->4, 1->3->4
输出:1->1->2->3->4->4
算法实现:
/** * Definition for singly-linked list. * public class ListNode { * public int val; * public ListNode next; * public ListNode(int x) { val = x; } * } */public class Solution{ public ListNode MergeTwoLists(ListNode l1, ListNode l2) { ListNode pHead = new ListNode(int.MaxValue); ListNode temp = pHead; while (l1 != null && l2 != null) { if (l1.val < l2.val) { temp.next = l1; l1 = l1.next; } else { temp.next = l2; l2 = l2.next; } temp = temp.next; } if (l1 != null) temp.next = l1; if (l2 != null) temp.next = l2; return pHead.next; }}
实验结果
相关图文:
转载地址:https://lsgogroup.blog.csdn.net/article/details/89359638 如侵犯您的版权,请留言回复原文章的地址,我们会给您删除此文章,给您带来不便请您谅解!
发表评论
最新留言
关注你微信了!
[***.104.42.241]2024年04月17日 15时21分58秒
关于作者
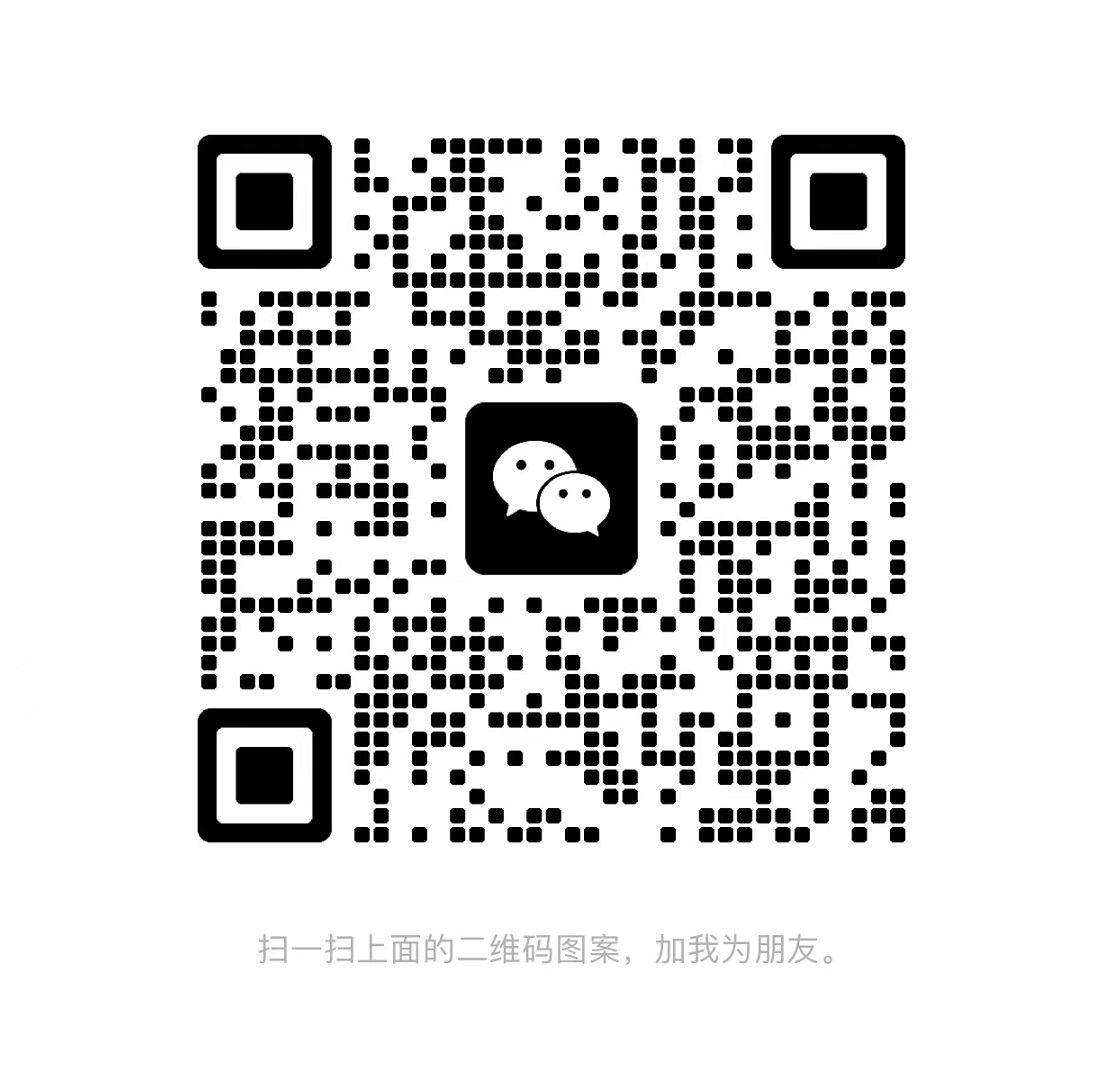
喝酒易醉,品茶养心,人生如梦,品茶悟道,何以解忧?唯有杜康!
-- 愿君每日到此一游!
推荐文章
RRT算法(快速拓展随机树)的Python实现
2019-04-30
强化学习(四) —— Actor-Critic演员评论家 & code
2019-04-30
RESTful API
2019-04-30
优化算法(四)——粒子群优化算法(PSO)
2019-04-30
数据在Oracle中的存储
2019-04-30
轨迹规划 trajectory planning
2019-04-30
AGV自动导引运输车
2019-04-30
Trie树(字典树)
2019-04-30
COMP7404 Machine Learing——KNN
2019-04-30
COMP7404 Machine Learing——SVM
2019-04-30
COMP7404 Machine Learing——ROC
2019-04-30