
sklearn 机器学习 Pipeline 模板
发布日期:2021-07-01 03:30:32
浏览次数:3
分类:技术文章
本文共 5207 字,大约阅读时间需要 17 分钟。
文章目录
使用 sklearn 的 pipeline 搭建机器学习的流程
本文例子为 参考1. 导入工具包
import numpy as npimport pandas as pd%matplotlib inlineimport matplotlib.pyplot as pltfrom sklearn.model_selection import train_test_splitfrom sklearn.model_selection import StratifiedShuffleSplitfrom sklearn.impute import SimpleImputerfrom sklearn.preprocessing import LabelEncoderfrom sklearn.preprocessing import OneHotEncoderfrom sklearn.preprocessing import LabelBinarizerfrom sklearn.base import BaseEstimator, TransformerMixinfrom sklearn.pipeline import Pipelinefrom sklearn.preprocessing import StandardScalerfrom sklearn.pipeline import FeatureUnionfrom sklearn.model_selection import GridSearchCVfrom sklearn.model_selection import cross_val_score
2. 读取数据
data = pd.read_csv("../competition/Employee_Satisfaction/train.csv")test = pd.read_csv("../competition/Employee_Satisfaction/test.csv")data.columns
Index(['id', 'last_evaluation', 'number_project', 'average_monthly_hours', 'time_spend_company', 'Work_accident', 'package', 'promotion_last_5years', 'division', 'salary', 'satisfaction_level'], dtype='object')
- 训练数据,标签分离
y = data['satisfaction_level']X = data.drop(['satisfaction_level'], axis=1)
3. 数字特征、文字特征分离
def num_cat_splitor(X): s = (X.dtypes == 'object') object_cols = list(s[s].index) # object_cols # ['package', 'division', 'salary'] num_cols = list(set(X.columns) - set(object_cols)) # num_cols # ['Work_accident', 'time_spend_company', 'promotion_last_5years', 'id', # 'average_monthly_hours', 'last_evaluation', 'number_project'] return num_cols, object_colsnum_cols, object_cols = num_cat_splitor(X)# print(num_cols)# print(object_cols)# X[object_cols].values
- 特征数值筛选器
class DataFrameSelector(BaseEstimator, TransformerMixin): def __init__(self, attribute_names): self.attribute_names = attribute_names def fit(self, X, y=None): return self def transform(self, X): return X[self.attribute_names].values
4. 数据处理Pipeline
- 数字特征
num_pipeline = Pipeline([ ('selector', DataFrameSelector(num_cols)), ('imputer', SimpleImputer(strategy="median")), ('std_scaler', StandardScaler()), ])
- 文字特征
cat_pipeline = Pipeline([ ('selector', DataFrameSelector(object_cols)), ('cat_encoder', OneHotEncoder(sparse=False)), ])
- 组合数字和文字特征
full_pipeline = FeatureUnion(transformer_list=[ ("num_pipeline", num_pipeline), ("cat_pipeline", cat_pipeline), ])X_prepared = full_pipeline.fit_transform(X)
5. 尝试不同的模型
from sklearn.ensemble import RandomForestRegressorforest_reg = RandomForestRegressor()forest_scores = cross_val_score(forest_reg,X_prepared,y, scoring='neg_mean_squared_error',cv=3)forest_rmse_scores = np.sqrt(-forest_scores)print(forest_rmse_scores)print(forest_rmse_scores.mean())print(forest_rmse_scores.std())
还可以尝试别的模型
6. 参数搜索
param_grid = [ { 'n_estimators' : [3,10,30,50,80],'max_features':[2,4,6,8]}, { 'bootstrap':[False], 'n_estimators' : [3,10],'max_features':[2,3,4]},]forest_reg = RandomForestRegressor()grid_search = GridSearchCV(forest_reg, param_grid, cv=5, scoring='neg_mean_squared_error')grid_search.fit(X_prepared,y)
- 最佳参数
grid_search.best_params_
- 最优模型
grid_search.best_estimator_
- 搜索结果
cv_result = grid_search.cv_results_for mean_score, params in zip(cv_result['mean_test_score'], cv_result['params']): print(np.sqrt(-mean_score), params)
0.2129252723367584 { 'max_features': 2, 'n_estimators': 3}0.19276874697889504 { 'max_features': 2, 'n_estimators': 10}0.1865548358477794 { 'max_features': 2, 'n_estimators': 30}.......
7. 特征重要性筛选
feature_importances = grid_search.best_estimator_.feature_importances_
- 选择前 k 个最重要的特征
k = 3def indices_of_top_k(arr, k): return np.sort(np.argpartition(np.array(arr), -k)[-k:])class TopFeatureSelector(BaseEstimator, TransformerMixin): def __init__(self, feature_importances, k): self.feature_importances = feature_importances self.k = k def fit(self, X, y=None): self.feature_indices_ = indices_of_top_k(self.feature_importances, self.k) return self def transform(self, X): return X[:, self.feature_indices_]
8. 最终完整Pipeline
prepare_select_and_predict_pipeline = Pipeline([ ('preparation', full_pipeline), ('feature_selection', TopFeatureSelector(feature_importances, k)), ('forst_reg', RandomForestRegressor())])
- 参数搜索
param_grid = [{ 'preparation__num_pipeline__imputer__strategy': ['mean', 'median', 'most_frequent'], 'feature_selection__k': list(range(5, len(feature_importances) + 1)), 'forst_reg__n_estimators' : [200,250,300,310,330], 'forst_reg__max_features':[2,4,6,8]}]grid_search_prep = GridSearchCV(prepare_select_and_predict_pipeline, param_grid, cv=10, scoring='neg_mean_squared_error', verbose=2, n_jobs=-1)
- 训练
grid_search_prep.fit(X,y)grid_search_prep.best_params_final_model = grid_search_prep.best_estimator_
- 预测
y_pred_test = final_model.predict(test)result = pd.DataFrame()result['id'] = test['id']result['satisfaction_level'] = y_pred_testresult.to_csv('rf_ML_pipeline.csv',index=False)
以上只是粗略的大体框架,还有很多细节,大家多指教!
我的CSDN
长按或扫码关注我的公众号(Michael阿明),一起加油、一起学习进步!
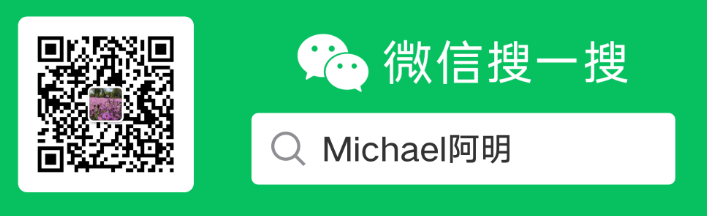
转载地址:https://michael.blog.csdn.net/article/details/107675895 如侵犯您的版权,请留言回复原文章的地址,我们会给您删除此文章,给您带来不便请您谅解!
发表评论
最新留言
感谢大佬
[***.8.128.20]2024年05月06日 02时18分43秒
关于作者
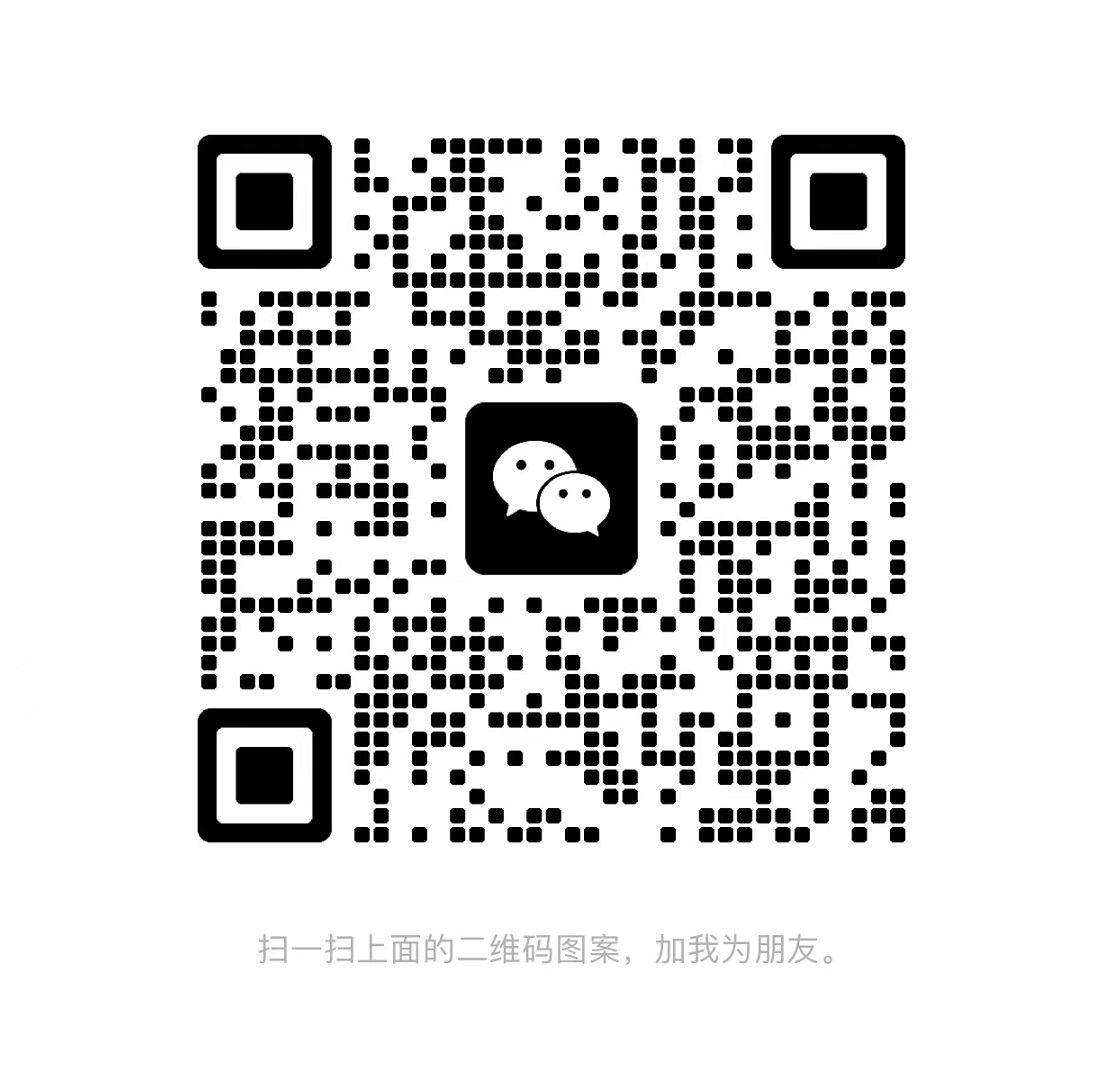
喝酒易醉,品茶养心,人生如梦,品茶悟道,何以解忧?唯有杜康!
-- 愿君每日到此一游!
推荐文章
“宇宙最强” IDE,Visual Studio 2019 正式发布
2019-05-03
每个程序员都该学习的5种开发语言
2019-05-03
都 9012了,该选择 Angular、React,还是Vue?
2019-05-03
WebApp 安全风险与防护课堂开课了!
2019-05-03
每个Web开发者都该了解的12条命令行
2019-05-03
十分钟教你理解TypeScript中的泛型
2019-05-03
2019 Vue开发指南:你都需要学点啥?
2019-05-03
如何编写更好的SQL查询:终极指南-第一部分
2019-05-03
WebApp 安全风险与防护课堂(第二讲)开课了!
2019-05-03
如何在Windows上使用Python进行开发
2019-05-03
如何使用JavaScript导入和导出Excel文件
2019-05-03
.NET Core 很酷,你不得不知!
2019-05-03
在 Windows 上使用 Python 进行 web 开发
2019-05-03
带你深入了解NPM——NPM初学者指南
2019-05-03
七天学会NodeJS——第一天
2019-05-03
初探Electron,从入门到实践
2019-05-03
不用JS,教你只用纯HTML做出几个实用网页效果
2019-05-03
随便写写
2019-05-03
spring 测试框架
2019-05-03
servelt filter listener 的生命周期
2019-05-03